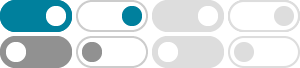
r - Best way to deal with heteroscedasticity? - Cross Validated
2015年4月19日 · The standard errors are wrong because of the heteroscedasticity. You can adjust the standard errors with the Huber-White sandwich estimator. That is what @GavinSimpson is doing in the linked SO thread. The heteroscedasticity does not make your linear model totally invalid. It primarily affects the standard errors.
regression - Understanding the causes and implication of ...
2020年11月30日 · $\begingroup$ @MichaelWebb : Gauss-Markov refers to the variance on a population level, i.e. under heteroskedasticity $\beta_{OLS}$ will "wiggle" more than $\beta_{GLS}$ when looking at multiple finite samples.
heteroscedasticity - Practically speaking, how do people handle …
As far as 'heteroscedasticity' I thought I was using the word in the ordinary sense: "a collection of random variables is heteroscedastic if there are sub-populations that have different variabilities from others. Here "variability" could be quantified by the variance or any other measure of statistical dispersion."-Wikipedia.
heteroscedasticity - What does having "constant variance" in a …
@Penquin_Knight has done a good job of showing what constant variance looks like by plotting the residuals of a model where homoscedasticity obtains against the fitted values. Heteroscedasticity can also possibly be detected in a plot of the raw data, or in a scale-location (also called spread-level) plot.
Heteroscedasticity in GLMM - Cross Validated
2020年5月8日 · I'm after some advice regarding heteroscedasticity in a residuals vs predicted plot. I have measured the length of a group of animals at birth and then at five subsequent time points into the future. As length is a continuous variable and I have random factors to account for, I have built a set of gaussian GLMM models in the glmmTMB package. I ...
Heteroscedasticity: When is it OK? - Cross Validated
2020年11月19日 · $\begingroup$ Leo Breiman's paper on the Two Cultures of prediction vs understanding is a good place to start. The classic approach to modeling, even before any data is gathered as would be done wrt many PhD dissertations, assumes a careful process of hypothesis generation leading to insight and understanding based on strict observance of all the …
terminology - Why are there two spellings of "heteroskedastic" or ...
2015年5月22日 · I frequently see both the spellings "heteroskedastic" and "heteroscedastic", and similarly for "homoscedastic" and "homoskedastic".
Dealing with Heteroscedasticity in ANOVA - Cross Validated
Note that without seeing data on your factors, the extent of heteroscedasticity can't be judged directly by us, but the distributions can't be less spiky. This would not go well in a comment, and the point that a big spike can only be transformed into another big spike arises commonly in this forum, so making the point emphatic may help others too.
heteroscedasticity - Dealing with Heteroskedasticity: when to use …
2019年10月10日 · I think current thinking is to not use transformations, but to either use an appropriate generalized linear model or to use a method like WLS. You might also look into methods that adjust for heteroscedasticity. For one-way designs there's Welch's anova. And R offers white-ajusted anova for heteroscedasticity.
heteroscedasticity - What are the consequences of having non …
2016年10月17日 · "Heteroscedasticity" makes it difficult to estimate the true standard deviation of the forecast errors. This can lead to confidence intervals that are too wide or too narrow (in particular they will be too narrow for out-of-sample predictions, if the variance of the errors is increasing over time).